Deciphering Design Principles for Antimicrobial-Nanobots Using AI
How can AI help combat the global crisis of antimicrobial resistance? Discover how advanced nanobot and AMP design offers a new approach.
Image what else AI could do for biology? also on the Ailurus website
Abstract: Antimicrobial peptides (AMPs) have significant potential to combat rising problems of antibiotic resistances, but their clinical use is limited due to issues such as toxicity and stability. Recent advancements have led to the development of "AMP-nanobots", which improve upon traditional AMPs by scaffolding them on nano structures. However, experimentally analyzing the structure of these designs is quite challenging. Here, we utilize AI-aided pipelines to analyze AMP-nanobots, focusing on distilling design principles. By screening a diverse combinatorial library, we provide insights into their structure-function relationships that could guide future design efforts of advanced antimicrobials to combat microbial resistance.
Introduction
Antibiotic resistances, and particularly, multi-drug resistance (MDR) are growing global health concerns. Antibiotic or antimicrobial resistance (AMR) occurs when microorganisms such as bacteria, viruses, fungi, and parasites evolve to resist the drugs that were once effective in treating infections. MDR, a subset of AMR, refers to the ability of these microorganisms to resist multiple antimicrobial drugs. These issues pose a significant threat to public health, making the discovery of new, effective antimicrobial agents imperative.
Antimicrobial peptides (AMPs) present a promising avenue for addressing these challenges. These peptides are versatile and potent molecules known for their broad-spectrum antimicrobial properties, with great potential across medical therapeutics, agricultural protection, and food preservation. However, it has generally been difficult to bring many AMPs to clinical use to combat AMR problems at a large scale. For instance, many AMPs exhibit toxic side effects on mammalian cells in the long term. Additionally, AMPs may be rapidly degraded by proteases in the body, reducing their effectiveness.
Recent advancements have paved a promising way for enhancing AMPs by constructing scaffolded AMP nano structures — nano-sized complexes where AMPs are attached to supportive frameworks to enhance their properties — which we refer to as AMP-nanobots hereafter.
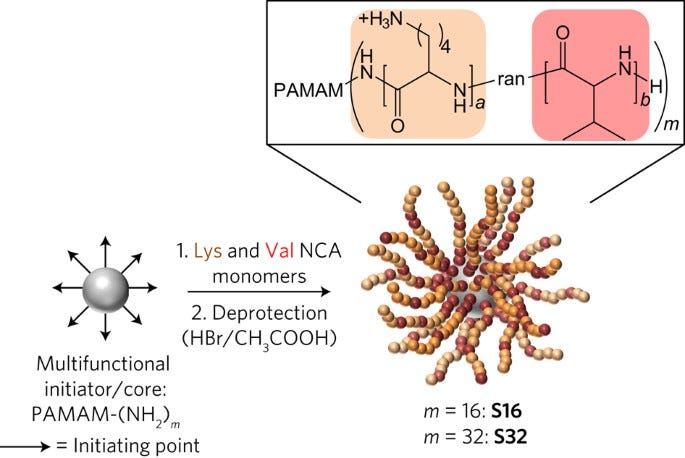
Early attempts include conjugating AMPs on dendritic chemical polymer cores, called structurally nanoengineered antimicrobial peptide polymers, or “SNAPP” by Lam et al. (2016).
In 2018, the iGEM Paris Bettencourt team developed the protein-scaffolded version of these nanobots, "STARCore". This approach makes these nanostructures fully programmable with biological materials, enabling precise control over the structures, and one of the first attempts at machine-learning based protein designs.
These nanoscale complexes retain antimicrobial properties while addressing key limitations of traditional AMPs, such as reducing their direct interaction with mammalian cell membranes. By integrating AMPs into structured frameworks, AMP-nanobots often offer a broader antimicrobial spectrum, with efficacy against gram-negative bacteria when equipped with AMPs traditionally only targeting gram-positive ones. This innovative approach presents AMP-nanobots as a promising solution to overcome the challenges that have historically limited AMP success.
Challenges in Designing AMP-Nanobots
Despite the progress, detailed guidelines to design AMP-nanobots are still lacking. Traditionally, rational design of proteins relies on accurate structure predictions and knowledge of structure-function relationships. Yet, these approaches are difficult to practice due to the limited knowledge of how AMP-nanobots work.
Firstly, the bactericidal mechanism of many AMPs remains largely elusive. Experimental methods such as X-ray crystallography, NMR, and Cryo-EM are particularly challenging for AMPs, due to their dynamic nature and small molecular weights. And computational efforts to apply molecular dynamics (MD) simulations are too costly to explore the enormous sequence space.
Additionally, it is also unclear why AMP-nanobots have broader spectrums. Observed in time-lapse microscopy, STARCore AMP nanobots killed bacteria cells in distinct patterns, with or without protein scaffolding. These results suggest significant mechanism shifts for unknown molecular reasons.
Here, we explore an AI-aided alternative to reverse engineer AMP-nanobots. Recent advances in protein structure prediction methods, such as AlphaFold and ESMFold, enable us to predict AMP structures and capture their dynamic properties. By leveraging AI-aided computational pipelines, we could investigate potential improvements in AMP activities and assess practical rules to create advanced AMP-nanobots for combating microbial threats.
Virtual Screening and Analysis of AMP-Nanobot Designs
In order to explore a large combinatorial library of AMP nanobot candidates, we need a method that provides accurate-enough structural predictions while using small-enough computational resources per design.
To achieve this, we employ the ESMFold module in the NVIDIA BioNemo service, a cutting-edge tool for protein structure prediction. ESMFold stands out for its ability to deliver highly accurate predictions comparable to those obtained using AlphaFold, one of the most renowned structure prediction algorithms. Moreover, it offers a significant advantage in terms of efficiency. Leveraging NVIDIA BioNemo's infrastructure for acceleration, it delivers short processing times without compromising the quality of the predicted structures.
Through virtual screening, we modeled the three-dimensional structures of AMP-nanobot designs swiftly, thus reducing reliance on expensive and time-consuming wet-lab experiments for structure determination. This allowed us to quickly assess which designs were most promising.
These modeled structures enable us to better understand the factors governing the performance of AMP-nanobots, providing insights into their potential functionality, stability, and microbial interactions. Additionally, the same computational pipeline could be used as a high-precision, efficient approach to identify the most promising AMP-nanobot design candidates for experimental validation, expediting the development process.
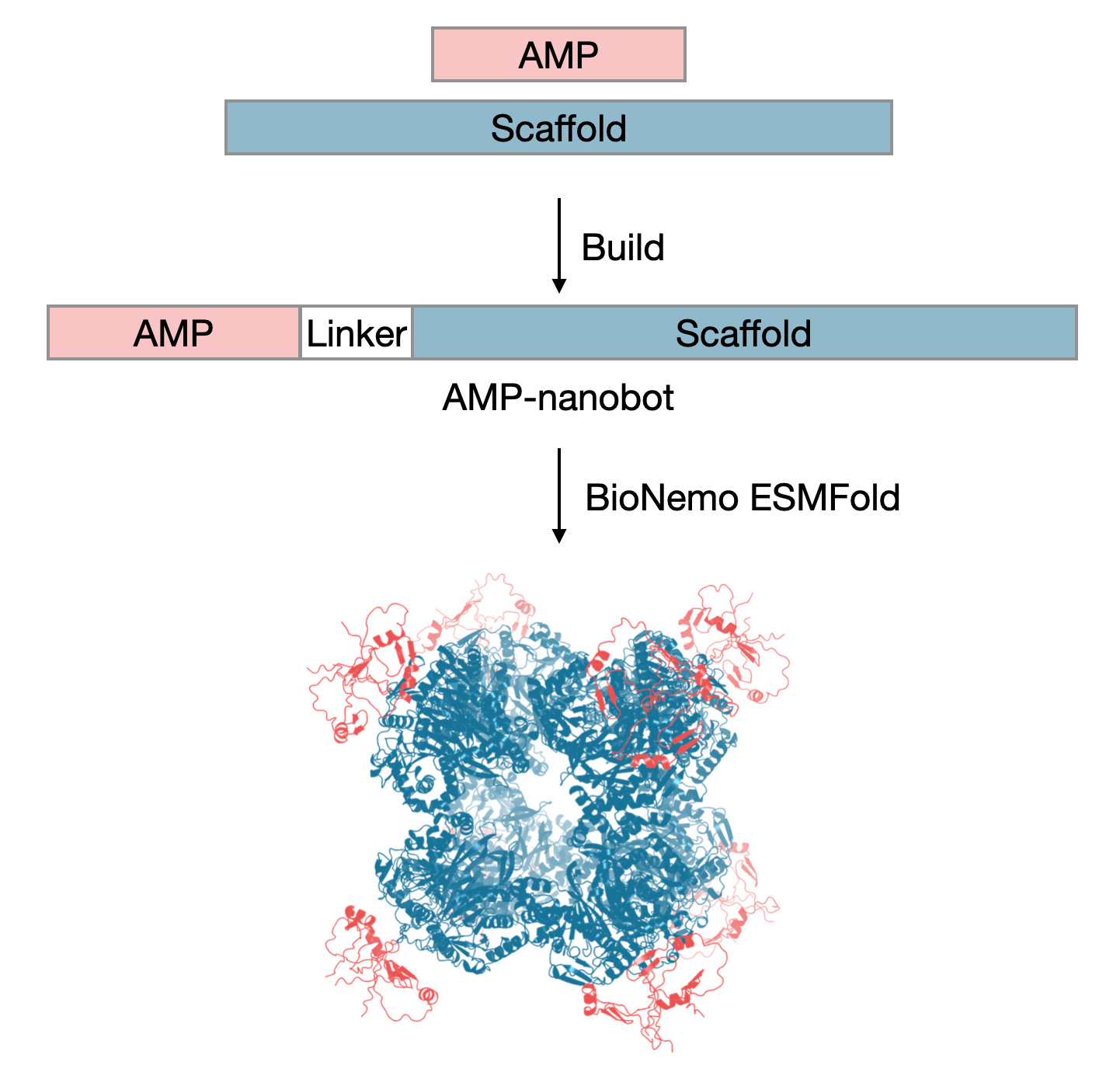
In this project, we curated a diverse collection of 28 AMPs and 15 scaffold proteins, providing a rich resource for creating novel AMP-nanobot configurations. To construct AMP nanobots in silico, we linked AMPs with scaffold proteins on either the N-terminal or C-terminal with a linker sequence. Scaffolds are homo-multimer proteins, such as the Dihydrolipoamide Acetyltransferase Core Domain (DLAT-C) from the pyruvate dehydrogenase complex. As a control, we also modeled AMPs alone without fusions ("blank"), and fused with a widely-used monomeric protein tag, Small Ubiquitin-like Modifier (SUMO). In total, we generated 952 unique designs for virtual screening analysis.
"Diffusing" Conformation of AMPs by Scaffolding
To understand the functional potential of AMP-nanobot designs, we analyzed their sequence and structure characteristics, focusing on properties influencing stability, efficacy, and antimicrobial activity, including:
Sequence Features: net charge, hydrophobicity, polar residue percentage, and isoelectric point (pI)
Structure Features: Solvent Accessible Surface Area (SASA), secondary structure elements (e.g., alpha-helices, beta-sheets), and pLDDT (predicted Local Distance Difference Test) scores.
The pLDDT values obtained from ESMFold provided valuable insights into AMP-nanobot functionality. The pLDDT scores estimate the overall confidence in the predicted structure. For instance, AMP-nanobots using Qbeta and MS2 scaffolds showed low pLDDT, consistent with the folding issues of these capsid proteins in ESMFold. Aside from these outliers, nearly all AMP-nanobot structures had high overall pLDDT values, suggesting reliable structural predictions.
We further analyzed the local pLDDT values in the AMP regions (AMP pLDDT). Generally, AMP pLDDT was lower in fusion proteins than in AMP alone. Notably, a strong fusion effect was universally observed for all AMPs fused with the monomeric SUMO tag. In well-folded predictions, lower pLDDT values often indicate a more dynamic or disordered structure, implying that fusion with additional proteins may generally increase AMP flexibility.
Despite the general decrease in AMP pLDDT in nanobot fusions, some combinations of multimeric scaffolds and AMPs had significantly higher AMP pLDDT than those fused with SUMO or other scaffolds. Many of them have been experimentally validated for strong, broader-spectrum antimicrobial activities (data not shown here). Detailed comparisons of predicted structures indicated that these increases were not merely a reversal of the fusion effect but represented the emergence of new conformations, revealing underlying changes in mechanisms and efficacy.
Together, we speculate that AMP-nanobots benefit from reduced toxicity and broadened spectrum by dramatically shifting the landscape of AMP conformational ensembles:
Increased AMP flexibility by scaffold fusion/conjugation
Induced conformations that are unstable in isolation
As a result, conformations toxic to mammalian hosts may be repressed, and hidden bactericidal mechanisms may emerge. Akin to a diffusion model for machine learning, nanostructured designs "diffuse" the functionality of AMPs, and "generate" novel properties. Experimental validation and screening methods could be established accordingly.
Discussion and Future Directions
By employing AI-aided methods, we were able to efficiently explore a large combinatorial library of AMP-nanobot candidates and gain deep insights into their structure-function relationships. Our results demonstrated significant changes in the conformational landscape caused by scaffold fusion, which may explain the improved stability, reduced toxicity, and broader antimicrobial activity.
The broader significance of this work lies in the novel reverse-engineering approach to using AI-aided structure predictions. Previous efforts in AI-aided protein design often started with a predetermined rationale or hypothesis and used pretrained models to generate sequences to meet those criteria. Instead, we started with a diverse set of experimentally validated designs and used AI models to conduct virtual screening in silico. This reverse approach enabled us to better understand the characteristics that contribute to the effectiveness of AMP-nanobots, providing valuable information to guide future design efforts.
These findings highlight the potential of integrating AI tools into the biotechnological development process, specifically for the discovery and optimization of antimicrobial agents. The ability to screen and optimize AMP-nanobot designs computationally provides a scalable and cost-effective way to accelerate the development of next-generation antimicrobials, which is crucial in the fight against antimicrobial resistance.
Acknowledge: Haotian Guo conceived this project, with workflow design by Haotian Guo and Bozitao Zhong. Bozitao Zhong completed the modeling, analysis, and data interpretation with Haotian Guo. We thank Nvidia for BioNeMo platform support, the 2018 iGEM Paris Bettencourt team for foundational work, and Ailurus team members for their reviews and feedback.
About Ailurus
Ailurus is a pioneering biocomputer company, programming biology as living smart devices, with products like PandaPure® that streamline protein expression and purification directly within cells, eliminating the need for columns or beads. Our mission is to make biology a general-purpose technology - easy to use and as accessible as modern computers.
For more information, visit: ailurus.bio
About iGEM Paris Bettencourt 2018
The iGEM Paris Bettencourt team is a multidisciplinary group from the Centre for Research and Interdisciplinarity (CRI) in Paris from 2007 to 2023. Competing in the iGEM competition since 2007 (initially as iGEM Paris), their work addresses a range of topics, from biosafety and 3D bioprinting to the human microbiome. The team emphasizes collaboration across biology, engineering, and design, consistently excelling with a Grand Prize win in 2013 and numerous finalist and special awards. In 2018, this team works on a project called "STARCore" to combat antimicrobial resistances.
For more information, visit: https://2018.igem.org/Team:Paris_Bettencourt
About Nvidia BioNeMo
NVIDIA BioNeMo is a generative AI platform designed to accelerate drug discovery by providing state-of-the-art biomolecular models and workflows. It enables researchers to customize and deploy AI models for tasks such as 3D protein structure prediction, de novo protein and small molecule generation, property predictions, and molecular docking. Accessible through cloud APIs or a user-friendly web interface, BioNeMo offers a seamless solution for integrating AI into drug discovery pipelines. The platform also includes the BioNeMo Framework, a collection of tools for designing and training biomolecular AI models, and NVIDIA NIM, a set of optimized microservices for AI models used in drug discovery.
For more information, visit: https://www.nvidia.com/en-us/clara/bionemo/